StyleGaussian: Instant 3D Style Transfer with Gaussian Splatting, Kunhao Liu, arXiv 2024
이번 리뷰는 개인적으로 여러 논문들을 보기 위해, 짧은 시간에 논문 파악하고 정리하는 연습을 목적으로 했습니다. 짧게 논문을 파악하고 싶은 독자들을 위한 글입니다.
요약
VGG output feature 로 3D Gaussian color를 표현하도록 모델링 후에, Style Image의 VGG Feature로 Switching 함으로써 Style Transfer수행. 3DGS parameter 모두 고정하고 color만 변형함
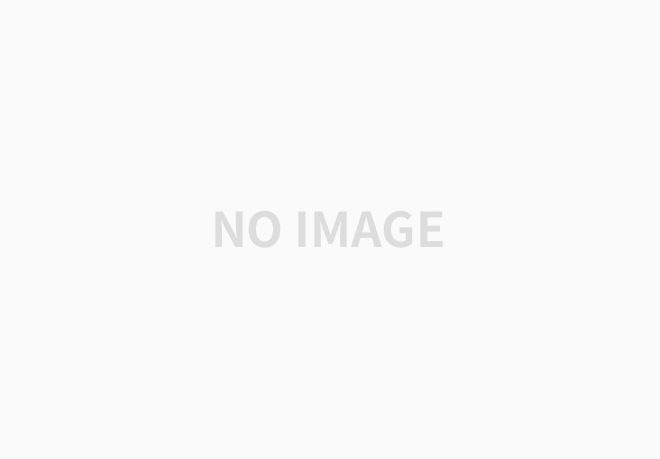
크게 Gaussian embedding, Style Transfer, RGB Decoding 3단계로 구성됨
Gaussian Embedding
VGG feature로 color를 표현
Vanila 3DGS Alpha Blending (α는 opacity) C=∑i∈Nciαii−1∏j=1(1−αj) StyleGaussian Alpha Blending : color대신 feature fi F=∑i∈Nfiwi,wi=αii−1∏j=1(1−αj) Loss function : Image의 VGG output Feature F_gt로 학습 {fp}Pp=argmin{fp}Pp∑|F−Fgt| Feature Dimension Reduction : 256(=F) -> 32(=F’) ; A,b는 learnable variables F=T(F′)=AF′+∑i∈Nbwi
Style Transfer
AdaIn으로 style feature transfer수행
Style을 VGG feature기반으로 transfer함
μ,σ : channel-wise 평균, 분산
Fs : style image의 VGG output
𝑓𝑇𝑝 : transformed feature ftp=σ(Fs)(fp−μ({fp}Pp)σ({fp}Pp))+μ(Fs)
RGB Decoding
Style Feature를 Rendering
Feature들을 CNN에 통과 시켜 Color를 획득하게 됨
이 때, KNN으로 K개의 인접한 gaussian들의 feature로 convolution함
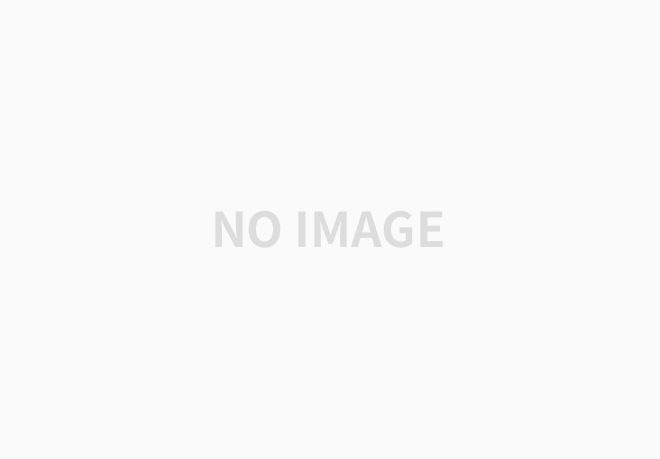
Loss함수 : style image의 feature와 유사해지도록 update ; RGB GT없음
Lc : feature map의 MSE, Ls : channel-wise feature 평균 표준편차의 MSE L=Lc+λLs Feature-to-color는 어떻게 학습? 디코더가 출력한 이미지를 VGG통과시킨 feature로 decoder를 학습하는 것이 아닐까 추측
실험 결과
link에서 영상으로 확인
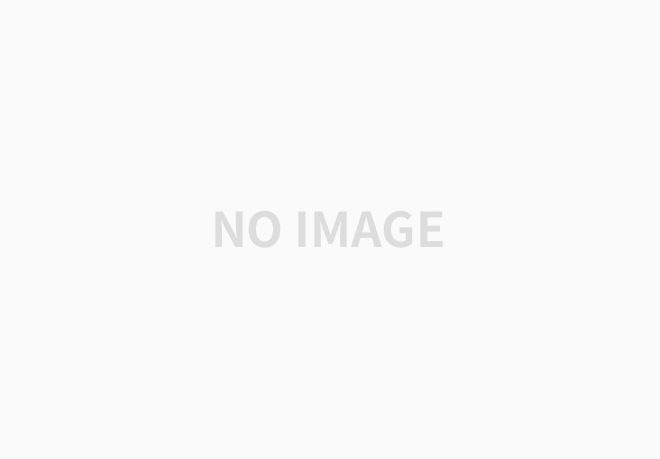
'3D-GS' 카테고리의 다른 글
[논문 리뷰] MVSGaussian (arXiv 2024) : Generalizable 모델 (0) | 2024.05.27 |
---|---|
[논문 리뷰] MVControl (arXiv 2024) : Image+Text to 3D (0) | 2024.05.22 |
[논문 리뷰] RadSplat (arXiv2024) : lightweight & 랜더링 속도 향상 (0) | 2024.04.30 |
[논문 리뷰] InstantSplat (arXiv 2024) : Pose-free GS (2) | 2024.04.24 |
[논문 리뷰] GPS-Gaussian (CVPR 2024) : Generalizable 모델 (0) | 2024.03.17 |
댓글